Insights
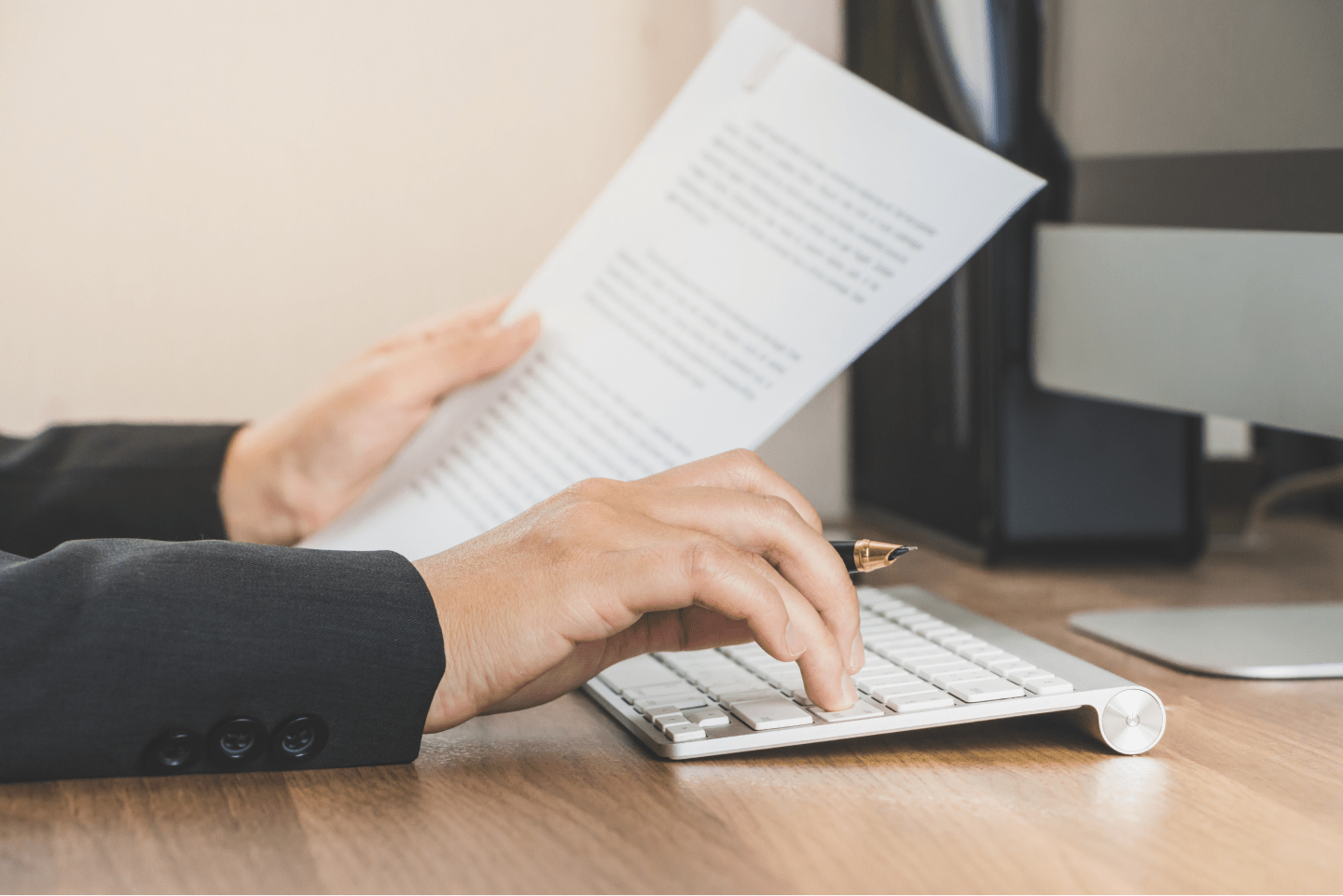
When is a simple model enough? How to handle structural uncertainty in cost-effectiveness models.
Sam Bryning, intern at Symmetron: ‘formally accounting for structural uncertainty in cost-effectiveness models makes research and decision making more robust’.
Cost-effectiveness models in healthcare attempt to capture the main features of clinical pathways that are often complex and uncertain. This involves defining a model structure and can involve making several simplifying assumptions. There may be many possible model structures to choose from, and it may be unclear which of these choices will produce more accurate predictions – this is referred to as structural uncertainty. Also, it is often unclear whether a complex model needs to be constructed to generate accurate results, or whether a simple model will suffice. Appropriately accounting for structural uncertainty is important for robust healthcare decision making and can aid the pursuit of reimbursement for new medicines and devices.
Sam Bryning completed a summer placement at Symmetron as part of his MSc. in Health Economics and Decision Modelling at the University of Sheffield. We spoke with him about his dissertation project on accounting for structural uncertainty in cost-effectiveness models.
Sam, why is structural uncertainty important?
It is important to fully consider structural uncertainty because, if not accounted for, it can bias the model results. Even if an intervention is predicted to be cost-effective, there may be considerable uncertainty around this prediction. This uncertainty could lead a healthcare payer to make a misinformed decision as to whether to reimburse a new medicine or device. Also, if the decision maker believes the model hasn’t captured the complexities of the decision problem in the model, they may ask for additional evidence or a new analysis, which requires additional investment by the manufacturer. Alternatively, the decision maker may just decide to not reimburse the new treatment, meaning that patients lose out, as well as the manufacturer. Appropriately quantifying this uncertainty can inform the value of future research and help identify the evidence that should be prioritised, or it may provide assurance that the structural uncertainty is not sufficient to change results and no further research is required.
What were the main objectives of the project?
My research attempts to compare the strengths and weaknesses of different approaches to account for structural uncertainty when modelling cost-effectiveness. This research will help to establish a method for accounting for structural uncertainty that balances the need for valid research and decision making, with the need to deliver models on time and that are simple to understand. Another key aim of the research was to find a method that could determine early in the reimbursement process whether a structurally complex model (which would take substantial time and money to develop) was worthwhile or if a simpler model could justifiably suffice.
Figure 1. The structure of a generic cost-effectiveness model. QALY, quality-adjusted life-year.
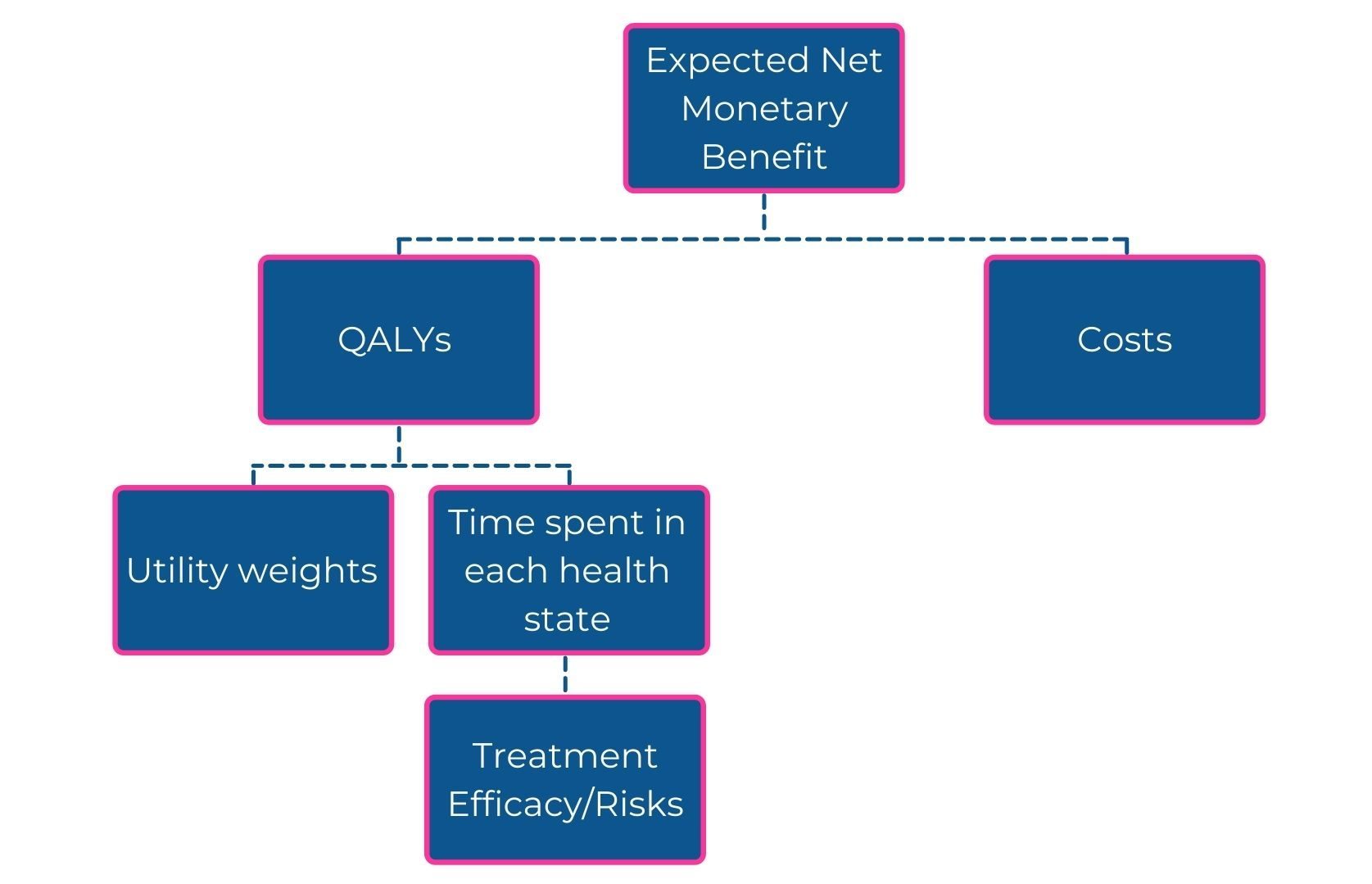
Why is this project needed?
Appropriately characterising structural uncertainty within cost-effectiveness models is challenging. Currently, the vast majority of cost-effectiveness analyses use scenario analysis to account for structural uncertainties or do not model them at all. Whilst scenario analysis is standard practice within the industry, it has methodological limitations and is not always possible to implement, due to the burden of modelling each scenario.
Further, the published methods for formally quantifying and responding to structural uncertainty are even more time-intensive, as they require developing at least one additional economic model, and weighting inputs or outputs using either data or input from experts. This is often unfeasible or goes against the goal of understanding if a more complicated model is required. The structural uncertainties that are most difficult to model are often the most important, so excluding them can undermine the robustness of results and decision-making.
This project aimed to research and implement a method for accounting for structural uncertainty that is both valid and practical. However, I needed to ensure the application of the method was not overly time intensive or computationally intensive. Like Symmetron researchers, I needed to fit the research to the project scope and timeline. I creatively adapted one of the published methods to fit within these constraints. I investigated whether the method could be applied to a small number of structural uncertainties using algorithms that are fast and easily implemented. I looked to make the published method more pragmatic, by introducing an alternative means to cater for correlations between parameters in the investigated approach.
How can structural uncertainty be formally accounted for?
There are various existing published methods to assess and account for structural uncertainty.
The simplest and crudest method, model averaging, attempts to combine the outputs of multiple models by assigning a weight to each one that reflects how likely it is to be ‘correct’. This weight is usually based on how well the model fits observed data, therefore its use is largely restricted to situations where we already have data available. Further, it requires developing all the different potentially correct models, which can be very time-intensive. There are also limitations to its validity.
Parameterisation is another approach encompassing a much broader range of methods and attempts to represent structural uncertainty as an additional parameter in the model. This parameter can indicate the probability that a given assumption is correct, analogous to the weighting that occurs during model averaging. The limitations of this method are similar to the model averaging method. It is more valid than model averaging, but also more time-intensive and more computationally intensive.
The discrepancy approach is a fundamentally different method that quantifies the magnitude of the structural error in a model. This approach involves conceptually breaking the model down into component parts known as ‘subfunctions’ (Figure 1): expected net monetary benefit is a function of costs and health benefits, which are in turn functions of health state costs/utilities, transition probabilities, and so forth.
For each subfunction, a new variable called a discrepancy term (also known as a structural error term) is added to reflect the potential structural uncertainty associated with the modelling of that subfunction. For example, a discrepancy term could be added to how we model health-related quality of life. By varying the value of the discrepancy term, we can highlight how important the way we model a given subfunction is to the evaluation’s results and consequently the decision problem.
Several discrepancy terms can be inserted into the model to account for different sources of structural uncertainty. Assessment of structural uncertainty for each subfunction is undertaken rather than the model as a whole, as making judgements about structural errors is much easier when only a simple process is involved (e.g. the cost incurred in a health state) than when the entire set of calculations is being considered.
To begin with, we can test a range of values for each discrepancy term, to see if a reduction of structural uncertainty would make any difference to our results. Then, if the discrepancy terms are determined to be important, they can be formally incorporated into the model, with the value of the discrepancy term informed by further research or expert opinion.
In my project, after undertaking a structured literature review to find out about the methods, I focused on using and enhancing the discrepancy approach and applying it to a case study that used a simple model structure. I modified the approach to ensure it was pragmatic and could be applied within the timescales of the project. An important part of my project was to ensure, that despite these modifications, the approach remained valid and robust. I validated the modified approach using a more complex version of the simple model.
What challenges have you encountered in this project?
One of the most challenging features of the work was the relatively underdeveloped literature and highly novel nature of the methods. As a result, there was little guidance or previous work to inform and validate the research. Fortunately, I have been able to carve a path through a thorough analysis of the literature, experimentation, and fruitful conversations with my colleagues at Symmetron.
What is the impact you expect to make?
My colleagues at Symmetron tell me that clients who desire an early-stage model may be interested in the modified discrepancy approach to inform them whether investment into a more complicated structure is worthwhile. Additionally, some clients may be interested in using the output of the modified discrepancy approach analysis to defend a simple model structure and demonstrate that a reduction of structural uncertainty would not change conclusions.
We plan to share this work with other health economists working within the industry, with the hope of inspiring others to improve the way that they account for structural uncertainty within cost-effectiveness models. In the long term, improvements to the way that structural uncertainty is handled would improve healthcare decision-making and patient outcomes.
How would you sum up your experience of completing an internship at Symmetron?
Although the research itself was challenging, the experience of working at Symmetron was very rewarding. The team was welcoming and always available to give advice or provide assistance, despite the challenges of a remote placement. This was valuable in providing a wider context to my research and helping me understand some of the challenges and problems encountered in practice. For the research itself, I had two supervisors who provided a close level of support, whether that be technical queries or guidance as to the general direction of the research. This support enabled me to pursue and complete an ambitious project that fulfilled my own personal objectives.
Similar Insights
Stay in touch
Subscribe to Symmetron and stay up to date with recent news and announcements.