Insights
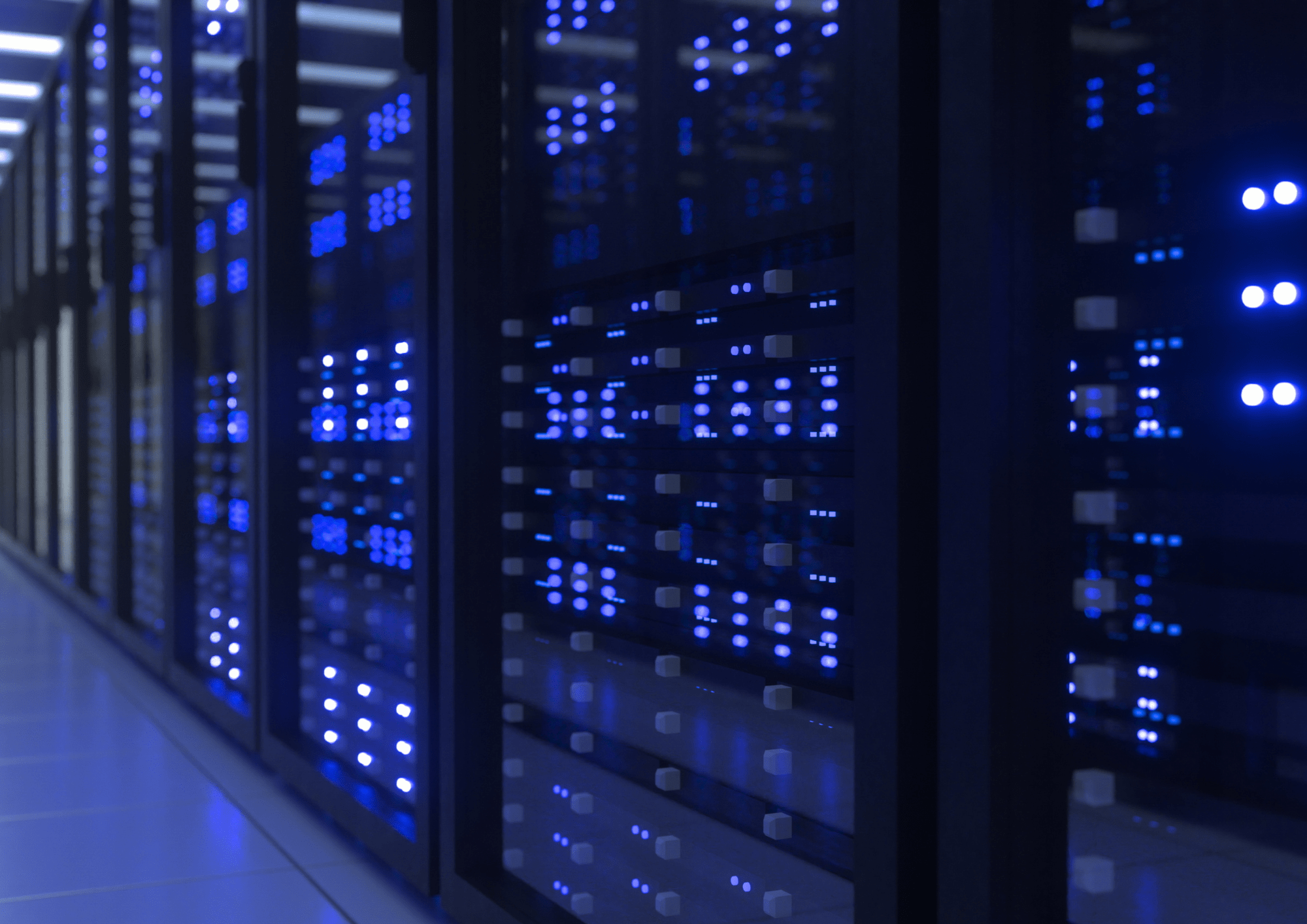
A cloud computing solution for improving the run-time of individual patient simulation models
What’s the longest time you left a health economic model running to complete a probabilistic analysis of 1,000 simulations? One of our senior health economists won our internal competition with a wild 5.5 days! In an effort to improve the significant simulation run-time we are all familiar with when it comes to models like health economics cost-effectiveness models, our team has successfully integrated the use of cloud computing. Our efforts have allowed us to improve the run-time of these models by up to 98% – from hours to minutes!
How did we achieve this and why is it a game changer for our efficiency when completing projects for our clients? This article will provide an overview of patient level simulations, how they are performed, and our game-changing approach to accelerating them. We illustrate how our solution allows us to maximise our efficiency and ensure seamless collaboration among all stakeholders involved in a health technology assessment (HTA) submission for a new health intervention.
What are patient-level simulations?
When modelling the cost-effectiveness of health technologies, it is important to consider which type of model is best suited for a specific intervention or condition as it can affect results and subsequently the validity and applicability of the model. For example, with cardiovascular conditions, it can be particularly important to capture any nuances of an individual patient’s profile and medical history as there can be interplay between associated risk factors and disease outcomes. Patient level simulation (PLS) approaches, such as a discrete event simulation (DES), can capture such nuances and model them comprehensively, whereby the outcomes are modelled for individual patients and then an average is taken across a sufficiently large sample of patients to produce a database for a cost-effectiveness model.
Why is model run-time an issue for a patient-level simulation?
When running a PLS, the number of patients simulated must be sufficiently high to ensure that the results have converged to produce a reliable model, so it is understandable that such models may become data-heavy and computationally demanding, commonly taking several hours to generate a single set of results. This is compounded when conducting a probabilistic sensitivity analysis (PSA) of parameter uncertainty simultaneously. PSA is important to include as it captures random variability in outcomes between clinically indistinguishable patients and variability between patients due to their characteristics. Assessing uncertainty of the input parameters using PSA has become increasingly important in HTA submissions in recent years with agencies such as National Institute for Health and Care Excellence and Canadian Agency for Drugs and Technologies in Health. As an example, if 1,000 patients and 1,000 probabilistic runs are required this means that 1,000,000 patient-runs will need to be performed (Figure 1).
Figure 1. The typical process of conducting probabilistic sensitivity analysis in patient level simulation
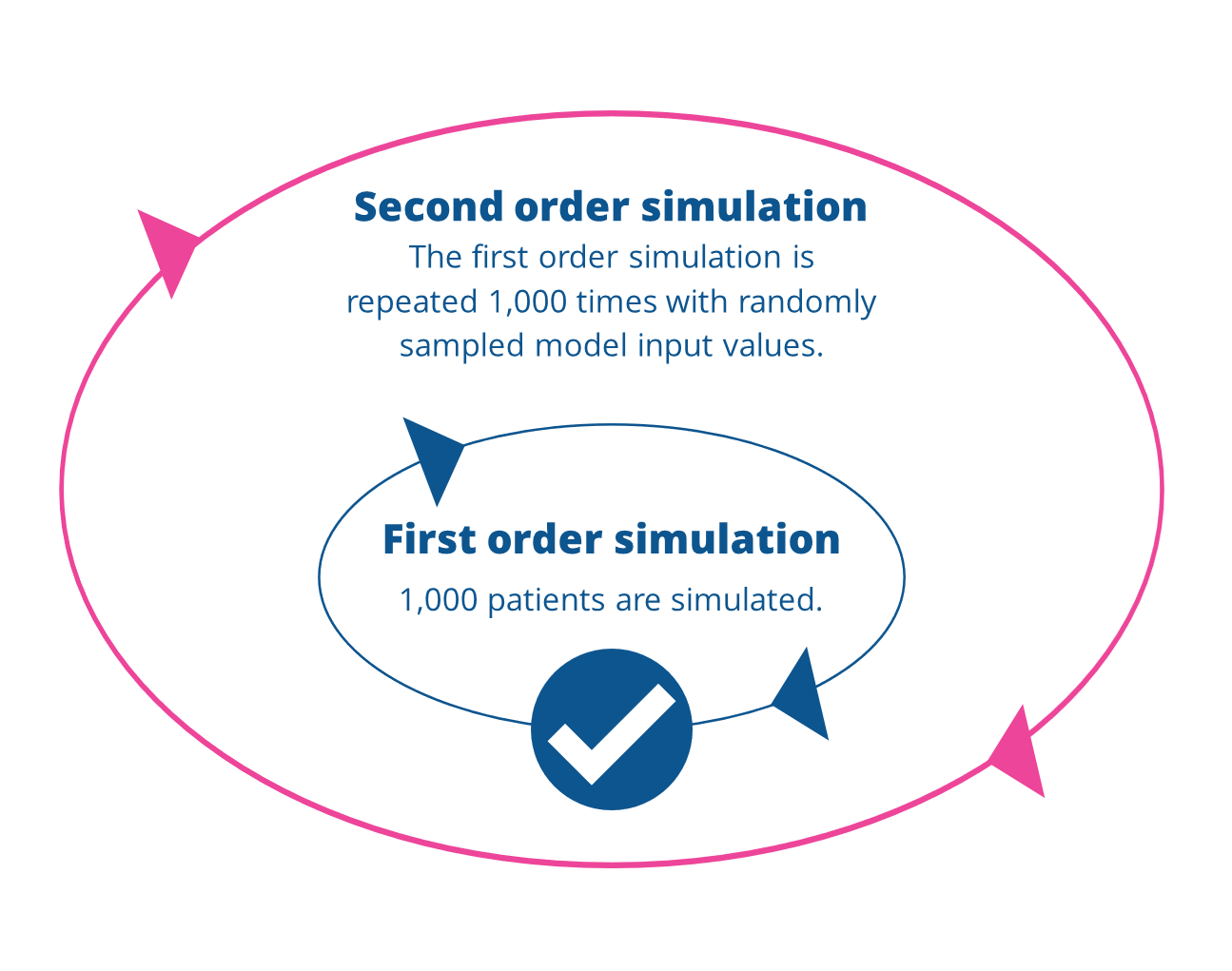
It is not surprising that such an analysis is likely to be computationally burdensome and could require many hours to run depending on the complexity of the model and the specifications of the computer. Therefore, the benefits of PLS must often be pragmatically balanced against a computational trade-off with the model run-time, as spending several hours generating one set of results is inefficient. Such an issue could be especially problematic for the HTA process where deadlines for submission and resubmission of material following clarification questions are often compressed and inflexible. If the run-time of PLS models can be reduced, then they can be considered a more practical choice for HTA modelling allowing health intervention manufacturers to leverage the benefits of PLS to calculate more accurate cost-effectiveness estimates for their technologies faster.
Our cloud computing solution to reduce simulation model run-time
At Symmetron, we have demonstrated how a retail cloud computing approach can be used to improve the run-time of these computationally burdensome health economic models, and we subsequently presented our results at ISPOR. Symmetron explored model run-time reduction in a DES model programmed in R to explore the cost-effectiveness of stroke prevention strategies in atrial fibrillation. Cloud computing was used to reduce the simulation model run-time via parallelisation, where an analysis is run on multiple central processing units (CPUs) simultaneously. The comparative computational power of run methods using parallelisation is graphically depicted in Figure 2.
Figure 2. Computational power of run methods at maximum capacity
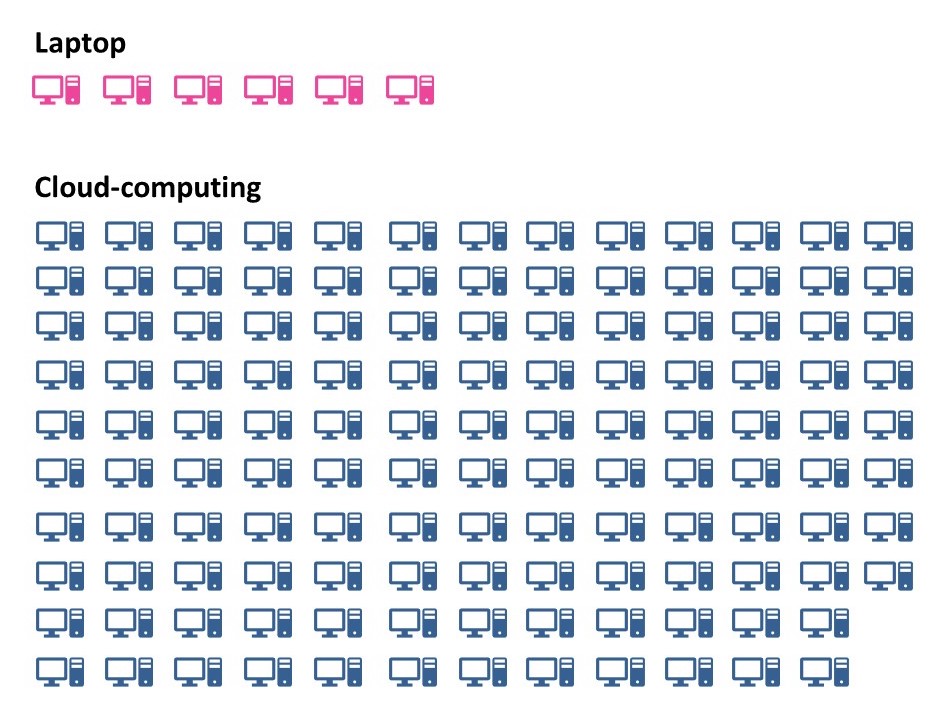
Analyses on a laptop utilising one and six CPUs were explored, as well as cloud computing analyses utilising eight, 32 and 128 CPUs. To assess the improvement in run-time, the DES model was executed using 1,000 patients for 1,000 PSA runs and the time taken was recorded. The total cost per analysis associated with each cloud computing approach was also recorded. The results of this analysis are shown in Table 1.
Figure 1. Run-time reduction strategies in a DES model
When using a laptop with no parallelisation the model took 4.3 hours to complete. This was reduced to 1.6 hours (a 63% reduction on run-time) by using a typical parallelisation approach with six CPUs. Using the cloud computing approach model runs with eight, 32 and 128 CPUs led to run-times of 52.4, 15.7 and 5.5 minutes (reductions of 80%, 94% and 98% respectively). The total cost associated with the cloud computing approaches ranged from USD 0.35-0.59 per analysis (Figure 3).
Figure 3. Run-time reduction strategies and the associated costs per run
.png)
How can our solution to improving simulation model run-time help you?
Our innovative cloud computing solution drastically improves the agility patient-level simulations, reducing the simulation model run-time from several hours to a few minutes. This allows us to exploit the power of these simulations in HTA submissions and resubmissions, despite the inevitably tight deadlines and complex mix of stakeholders who must work together smoothly to ensure the best outcome. In addition to saving precious time and resources, our approach will help bring life-saving treatments to patients faster.
Are there any other methods or approaches from other disciplines that you think could be used to innovate the field of health economics? Let us know at hello@symmetron.net!
You can find out more about our service offerings and reimbursement success stories on our website. Please contact us here to discuss how Symmetron can add value to your work.
Similar Insights
Stay in touch
Subscribe to Symmetron and stay up to date with recent news and announcements.